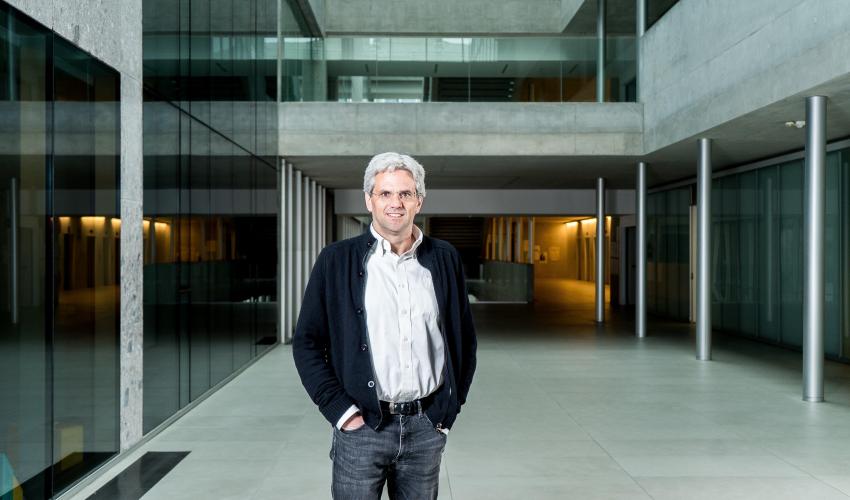
Everyone is looking for answers from AI. But the key lies in the questions
MACHINES ARE VERY USEFUL FOR FINDING SIMILAR PATTERNS IN HUGE AMOUNTS OF DATA, BUT THEY LACK ABSTRACT REASONING, A DISTINCTIVE QUALITY OF THE HUMAN MIND. IN THE FUTURE, HUMANMACHINE INTERACTION WILL LEVERAGE THEIR RESPECTIVE STRENGTHS, EXPLAINS IGOR PRUENSTER, DIRECTOR OF BIDSA, THE RESEARCH CENTER THAT COMBINES EXPERTISE IN STATISTICS, COMPUTER SCIENCE AND DATA SCIENCEBringing together various disciplines such as statistics, computer science, mathematics and social sciences, and applying computational analysis tools to analyze large amounts of data and create models of complex phenomena. This is the research mission the recently established Bocconi Institute of Data Science & Analytics Institute (BIDSA) is pursuing. The founding director of the research center, Professor Igor Pruenster, Full Professor of Statistics and President of the International Society for Bayesian Analysis, does not share the current fad for using the word ‘AI’ as much as you can. “As an academic, I care a lot about the definition of concepts, and the word artificial intelligence is anything but precise; it is a very large box filled with many different concepts and tools,” he explains. “The use of the notion of intelligence is potentially misleading if we have human intelligence as a reference because an AI system works very differently. Today we are mostly dealing with systems that perform precise prediction or classification tasks by applying statistical and mathematical techniques to datasets, in order to identify patterns or recurrences. It is not difficult to imagine that if you are able to store the online browsing data of a large portion of the population, you are capable of predicting the online behavior of each of us. We are much less unique than you’d think. Just type a question on a search engine to see that it auto-completes because many others before us have already asked the same question”.
What are the truly innovative aspects of AI, then?
The questions we are asking are not new, but the scale makes all the difference. Analyzing thousands of ultrasounds for a pattern matching is a relatively simple operation for a computer, but one for which human intelligence is extremely fallacious. Even the most experienced doctor relies above all on his own experience which, however extensive, remains anecdotal. This does not imply that the doctor's intervention is redundant, but that they will have a scientifically more solid decision-making support. It is more difficult for machines to imagine something that is not yet there. Already Turing, as he was trying to decipher the Enigma code at Bletchley Park during World War II, dealt with the simple but formidable problem of estimating the probability of discovering a new species. In their context, this corresponded to the appearance of a new grouping of letters in the intercepted encrypted dispatches. Today the interest in this type of research has grown significantly. Just think of genomics and the importance of estimating the probability of sequencing new genes or that a new variant of SARS-CoV-2 will emerge. The common view at the moment is that computers will not be able to emulate humans in the capacity of abstract reasoning in the near future. The most profitable development of artificial intelligence will therefore be in human-machine interaction by leveraging their respective strengths.
Today AI is used in every context. So does studying it means you have to become an expert in everything?
The digital revolution has changed research in almost every area, primarily thanks to the wide availability of data and computational potential. As a consequence, a modern researcher must have solid background in computer science, statistics and mathematics, as well as being an expert in their own field, the so-called domain-specific knowledge. Even those who develop methods for learning from data today, which are typically labeled as AI, machine learning or data science, are required to be more interdisciplinary than in the past. However, the trade-off between depth and breadth hasn't gone away and there’s a constant search for balance between the two. The risk of becoming all-purpose researchers is always around the corner. For this reason, I always recommend PhD programs in Statistics and Computer Science to students interested in AI and data science who intend to undertake research careers in universities or industry. This allows them to acquire a well-defined specialization, a natural home, and constitutes a springboard for subsequently broadening their spectrum of interests. As it has happened to many of my students who now work for Google or Amazon, in the industry they are placed in composite teams whose members have very different skills to the point that interdisciplinarity is automatically achieved at the team level. It is no coincidence that inside BIDSA we have set up four units, the Artificial Intelligence Lab (ArtLab), Bayesian Learning Lab (BayesLab), Data and Marketing Insights (DMI), and Blockchain Initiative, to give our community of researchers and students the opportunity to aggregate in smaller groups around specific subjects, thus grafting a vertical model onto the horizontal one that already existed”.
Does the interdisciplinary approach shape a new subject and a new way of doing research?
Yes. In this sense I think Michael Jordan, who is not the basketball champion but a Berkeley professor or rather, as the magazine Science dubbed him, "the Michael Jordan of Computer Science", is right. Michael, who is often visiting professor at Bocconi and gave one of the talks at the inaugural BIDSA conference, argues that we are witnessing the birth of a new branch of engineering that is based on data and learning. In fact, AI is based on well-rooted ideas: data, uncertainty, information, algorithms, inference, optimization. These are concepts that have been studied in depth by various disciplines such as statistics, applied mathematics and computer science. In the 1990s we began to mix these components and today's novelty, thanks to current level of computational resources, lies in doing so on a large scale with a direct impact on society. This will be a new branch of data engineering and learning, in the same way that chemical engineering is founded on chemistry but analyzes its application in industrial processes. The other relevant novelty is that, for the first time, we are starting from data provided by people about people. To quote Jordan again, it will be the first branch of engineering centered around people.
What are the frontiers in AI research?
Looking at the fundamentals, I expect that issues such as uncertainty quantification, robustness and interpretability to gain more and more prominence. The quantification of uncertainty is a cornerstone of statistics but, as it conjoined with computer science, this was a bit lost. There is this somewhat naïve idea that, as the quantity of data increases, uncertainty vanishes. In most cases this is not the case. Probably most have heard of ChatGPT; think how much more reliable it would be if it gave replies associated with a measure of confidence about the various parts of the answer given. The second theme is the robustness of the modeling. Would the results change by changing the parameters of the model or perturbing the data? At present, the differences would be significant and this is a limitation of the models. The third factor is the interpretability of results. One of the major shortcomings of modern deep learning models is that they provide predictions that are often accurate, yet it remains very difficult, if not impossible, to understand how they arrived at their conclusion. Knowing that you have optimized an objective function is enough to decide what film to suggest a user, but it is not always enough for deciding on a medical therapy. Understanding is always important, in my opinion.
What are the fields of application of all this that you explore within BIDSA?
We have several ERC-funded projects, one on the quantification of uncertainty and others at the intersection of robustness and interpretability. Further projects deal with medical networks, language processing technologies incorporating demographic factors as well as pure mathematics on optimal transport, an abstract problem that has numerous applications today. And then there is a group of economists studying decision theory, a subject that does not yet play an important role in AI but which will increasingly come to the fore. Today, in fact, the focus of research and applications of AI is concentrated on data and on the best decision for a single individual, while federated learning, i.e. the interactions between individuals and between them and the context, is still little explored.
by Emanuele Elli
Translated by Alex Foti