
Data, the evolution of the species
IN THE BEGINNING THERE WERE THE NUMBERS OF LIKES OR POLLS, THEN THE REVOLUTION BEGAN THROUGH WORD EMBEDDING AND COMPUTER VISION. THANKS TO NEUROSCIENCE AND DEEP LEARNING, ORGANIZATIONS HAVE THUS BEGUN TO USE TEXTUAL, VISUAL, AND MORE RECENTLY AUDIO DATA THAT RETURN USEFUL INFORMATION TO SEGMENT THE POPULATION. THE FUTURE? ANALYZING EMOTIONS, A MARKET THAT IS EXPECTED TO BE WORTH $ 56 BILLION IN 2024by Gaia Rubera, Amplifon Chair in Customer Science and Director of the Department of Marketing, Bocconi University
It goes without saying that data is the most important resource in the data-driven economy. Over the years, the type of data available to organizations, our models with which to analyze it, and the knowledge we gain from it, have profoundly changed.
Initially, there were numbers, from a variety of sources: e.g., loyalty cards, likes and shares on social networks. This data, coupled with machine learning models, provided us with a more refined understanding of customer wants and preferences. Firms like Amazon and Google built their fortune around it. Most importantly, and alarmingly, organizations could correlate numbers to sensitive information. For instance, Target learned that women who buy hand lotion and vitamins are most likely to be pregnant. Cambridge Analytica learned that a simple like to a post about The Addams Family reveals that one is neurotic.
However, if you think of human beings, numbers are just a small piece of data that we process daily. We also receive data from discussing, reading, and our senses of sight and hearing, for example. In the last decade, advancements in computer science augmented numbers with “unstructured data”, i.e., text, images, and audio data.
Unknown to most at the time, a revolution happened at Google around 2013. Until then, words were treated as atomic units, namely as indices in a vocabulary with no relationship between them. Drawing from linguistic theory, Google scientists developed a neural network model – called word embedding – that represents words as vectors, where closer vectors indicate more semantically similar words. For example, the vector for “coffee” is close to the vector for “tea” but further from the vector for “soccer”. Do you remember Google Translate a decade ago? Hard to use. Have you tried it nowadays? It can instantly translate between any pair of languages. All this because of word embedding models. The implications for our understanding of society are equally remarkable. We can now infer historical stereotypes and assess changes to these stereotypes over time. For instance, by analyzing news outlets, Garg and colleagues (2018) observed that the vector for “emotional” gets closer to the vector for “woman” over time, until it becomes a word to describe women in a pejorative sense. Similarly, firms can mine textual data from social networks and use word embedding to track how consumers’ perceptions change over time or in response to specific events, such as marketing campaigns, scandals, or new product introductions.
Professor Gaia Rubera is one of the protagonist of the first episode of the new Story Scanner podcast, with further insight and analysis on the topics headlining viaSarfatti25 magazine. In this episode we discuss Big Data and Data-Driven Innovation.
Over a similar time period, another stunning advancement happened in the field of computer vision. At an annual competition for recognizing objects in images, scientists from the University of Toronto presented AlexNet, a deep learning architecture that smoked all competing algorithms, dropping the error rate from 26.2% (the closest competitor) to an astonishing 15.3%. What was so special about AlexNet? It translated neuroscience models of how vision occurs in the human brain into a computer science model. It is a monument to the spectacular achievements of computer science meeting neuroscience. Today, the offspring of AlexNet are everywhere, from models analyzing MRI scans to detect early signals of cancer to Disney tracking the facial expressions of moviegoers to predict movie sales, to logo recognition algorithms identifying Instagram images of consumers using a brand’s products. Through these images, firms can now conduct ethnographic studies on millions of consumers – rather than on a few dozen, as in the past.
Among the many computer vision applications, one is particularly lucrative, yet unsettled: emotion detection, a market projected to be worth $56 billion in 2024. Current applications largely rely on facial expressions. However, cognitive and neuroscientists report that multimodal representations (e.g., face and voice) yield faster and more accurate emotion judgments than unimodal presentations (e.g., face only) (Klasen et al. 2012). Vocal features such as pitch, tone, and loudness provide helpful clues that human beings use to detect emotions. The next frontier is, hence, the development of multimodal models that combine visual and audio data. Such models could pave the way to a different type of segmentation, no longer based on stable demo-psychographic characteristics, product usage, or purchase patterns. Rather, we could segment, and target, consumers according to their mood in the specific moment they interact with an organization. A quantum leap from the meager information that we could gather from surveys or focus groups just a few years ago.
Continue reading here the cover story of the magazine viaSarfatti25
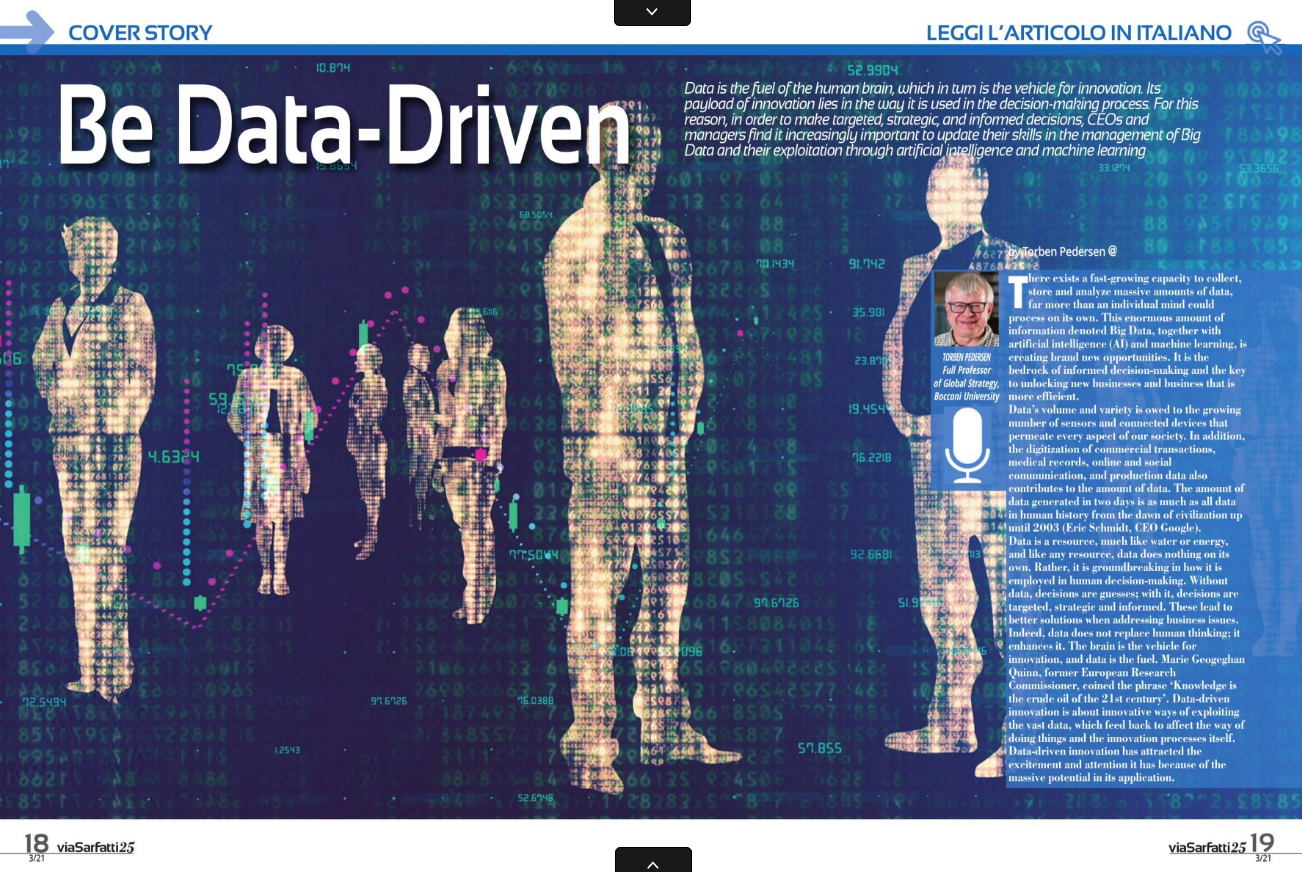